Predictive Analytics: benefits and perspectives of the market
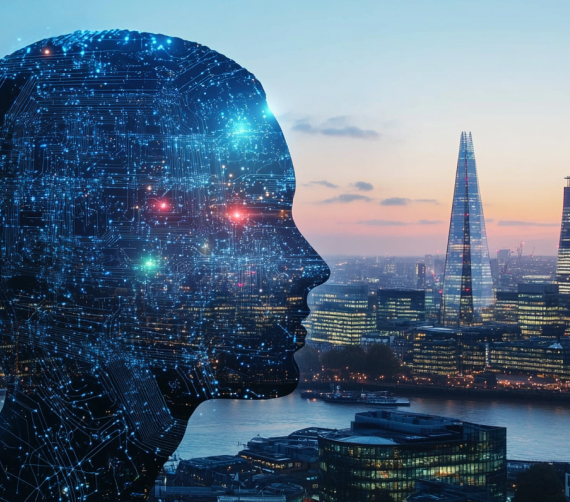
The future of most commercial and noncommercial sectors is tightly connected with recent technological innovations. International corporations invest billions of dollars into Artificial Intelligence, Big Data, and Machine Learning solutions. This said, the sphere of Predictive Analytics (PA) serves as a catalyst for capitalizing on the recent innovations. In 2023, the global PA market reached $10.5 billion, according to recent market research. The estimated value of the sector by 2028 is $23.9 billion – analysts expect significant growth of the market. What is “Predictive Analytics” and why is the sphere important for today’s businesses?
Predictive Analytics: The notion and basic principles
If to start with “analytics”, the process is understood as the systematic numerical analysis of data and statistics to find somemeaningful patterns, and the usage of these patterns for effective decision making. Driven by AI, Predictive Analytics is the secondlevel in the analytics process hierarchy.
In simple words, PA mechanisms are responsible for predicting what will happen in the future within a certain field. PredictiveAnalytics is understood as a specific class of data analysis methods that relates to the prediction of objects/subjects’ future behaviormodels. The basic principles behind PA originate from the 1940s, but recent technologies like AI, Big Data, and ML have opened new horizons for PA practitioners. The Predictive Analytics process is based on the four key constituents. The first two stages, formally speaking, precede the PA process, but doing analytics is impossible without them.
1. Goal setting: The goal setting stage together with hypothesis statement (the possibility to predict desired events on the basis of given data) lay the ground for the next steps.
2. Data collection: Data is the basis of every statistical analysis, including the Machine Learning approach. There are two critical characteristics which distinguish a successful data collection stage: data volume (dataset depth) and data quality. Big Data technology provides data practitioners with powerful instruments that make acquiring sufficient data volumes significantly more feasible.
3. Exploratory data analysis: Data itself is insufficient for making predictions. You need to implement appropriate approaches to discover interdependencies inside modern day data volumes. According to IDC, the annual growth of data being stored is 20.4%, and the total volume of data is expected to reach 8.9 zettabytes by 2024. Artificial Intelligence helps not get lost within such volumes of raw data by unraveling hidden correlations.
4. Predictive modeling: The closing chapter of the process is the recognition of data insights. This stage implies the construction of a mathematical predictive model to execute the necessary tasks. Machine learning technology utilization is the current trend at this stage.
5 top open source use cases of Predictive Analytics: Brief overview
So, let’s take a sneak peek into several success stories in which harnessing the power of Predictive Analytics has brought about great results for business customers.
1. Delivering an ML-based algorithm for predicting NBA games results.
Challenge: A client needed an ML-based model to predict the chances of each team to win the next NBA game. Strategy: The model was based on a recurrent neural network trained on a huge masses of NBA matches results.
Solution: The RNN-based model shows high forecast accuracy. The developers plan to test a model based on the temporalconvolutional network, which provides image and video recognition to improve the forecast results.
Results: The current modelaccuracy is higher than 80%.
2. Ensuring AI-backed services for effective asset management.
Challenge: Catana Capital needed ahighly effective service for accurate trading predictions and asset management.
Strategy: The service is based on the Big Data, AI, andPredictive Analytics methods, analyzing thousands of news, financial articles, blog posts, and other information to get the mostcomplete picture of the market.
Solution: The service uses quotes from more than 45 thousand shares to get the most accurate forecasts of further price movements.
Results: As of now, this AI-driven Catana Capital service is in high demand among traders worldwide.
3. Implementing biometricverification based on voice data
Challenge: Call-centers needed an effective and secure authentication system, resistant tocyberattacks and expected to be convenient for users.
Strategy: The voice-based authentication system was set for furtherimplementation. The system included the database of stored voice prints created to recognize speakers’ voices.
Solution: The system comprised a neural network to ensure that a certain voice corresponded with a stored voiceprint of a certainperson.
Result: Business customers received the voice-based authentication system with high security to reduce the verification timeand increase the authorization process efficiency.
4. Enabling cash flow optimization in ATM network system
Challenge: When operated manually or semi-automatically, the ATM network faced some significant hardships related to overly large expensesand imprecise monitoring results. The network required the estimation of the optimal amount of funds for cash collection.
Strategy: The PA methods were leveraged to predict the exact daily cash withdrawal amount and define the optimal cash flow.
Solution: Based on the bank ATM data, the daily cash withdrawal amount was forecasted with maximum error indexes of 0.01-3.5%while utilizing cash flow Predictive Analytics algorithms. Results: Cash use efficiency increased by 15-40%, and the downtime of ATMmachines dropped to 0.2%.
5. Providing accurate forecasting of electricity consumption rates
Challenge: An energycompany needed an effective, ML-based electricity consumption model. Furthermore, the task included building up a forecastingsystem that would enable a company to plan its purchase volume on the energy exchange.
Strategy: Recurrent neural networks wereapplied for building a forecasting system with the maximum preciseness index.
Solution: On the basis of open source New York City hourly energy consumption data and temperature fluctuation, the forecastingmodel for 2 days’ consumption rates was constructed.
Result: The forecasting ML-based system delivered to the business customerhas a preciseness index of 96.4-99.5%.